Animals not only capture attention, but they are monitored in an ongoing manner by a high-level, category-specialized system that was shaped by ancestral selection pressures, not general learning processes.
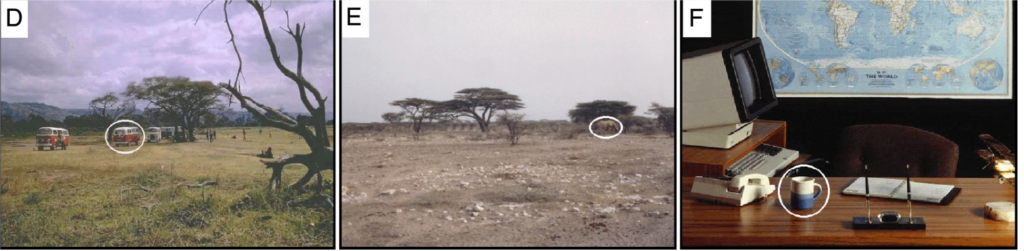
How often were changes detected? Elephant 100% of the time; red minivan 72%; mug 67%
Category-Specific Attention for Animals Reflects Ancestral Priorities, Not Expertise by Joshua New, Leda Cosmides, & John Tooby, PNAS 2007
Through ancestors’ eyes
Experiments show that the visual priorities of our hunter-gatherer ancestors are embedded in the modern brain. What our eyes look at is guided by brain mechanisms that pick out some portions of a scene over others. Since keeping an eye on predators and prey was important during our evolution, Joshua New and colleagues investigated whether animals, both human and otherwise, are more likely to draw visual attention spontaneously. The researchers showed subjects pairs of photographs of natural scenes in rapid alternation, with the second photograph including a single change. As predicted, subjects were faster and more accurate detecting changes involving animals than inanimate objects. If experience were producing this attentional bias for animals, then people should also be good at detecting changes to vehicles—they have been trained all their lives, as drivers and pedestrians, to monitor vehicles for sudden, life-or-death changes in trajectory. Yet they were much slower in detecting changes to vehicles than to more rarely experienced animal species, indicating that learning is not the source of this difference. The bias for animals, the authors conclude, is like the appendix—present in modern humans because it was useful for our ancestors, even if useless now. (From the PNAS press alert.)
(Historical note: Darwin used the existence of now vestigial organs, like the appendix, in arguing against creationism. Is this research relevant to debates about “intelligent design”? See below)
For brief accounts, see The Economist and Andrea Estrada’s UCSB press release.
Detecting changes to animals
These experiments used a change detection paradigm, which is famous for eliciting “change blindness”—the failure to see even major changes to a scene. But change blindness is attenuated for nonhuman animals and people. That is, changes to animals or people in a scene are detected quickly and accurately, even if changes to buildings, plants, tools, or vehicles are not. For an online demonstration of how it works, click here.

Change detection paradigm. The scenes alternate–you are asked to click as soon as you see what, if anything, is changing. In this example, the steeple disappaears and reappears.
Deep background: What did we do, what did we find, and how does this research depart from usual approaches to visual attention?
Research on visual attention has been almost untouched by behavioral ecology. By asking questions arising from behavioral ecology—specifically from areas dealing with adaptations to the presence of prey and predators in a species’ habitat—we were led to look for a mechanism of visual attention that was previously unknown. The research we present is a departure from almost all previous studies of human visual attention in three ways:
- Whereas most research on visual attention focuses on low-level features, ours presents evidence of an attentional system that is category-specific for animals. This is the first high-level, category-specific attentional system found for a stimulus class other than humans.
- This system is independent of fast recognition, operating on different principles. In addition to causing attentional capture, it monitors animals in an ongoing fashion for changes in their state and location.
- We present evidence that this animal monitoring system originates in phylogeny rather than ontogeny. The only other stimulus class known to elicit category-specific attentional monitoring is humans, but effects of phylogeny and ontogeny cannot be dissociated for that category. So ours is the first evidence for phylogenetic shaping of a category-specific attentional monitoring system.
Category specificity
We derived the hypothesis of an animal monitoring system by considering what impact an evolutionary history of foraging and predation should have had on the priorities built into human visual attention. To test this hypothesis, we used the change detection paradigm, in which subjects are asked to spot the difference between two rapidly alternating natural scenes. This paradigm is famous for eliciting change blindness—a condition in which observers are unaware that the scene is changing, even when major changes are introduced. (E.g., whole buildings can repeatedly appear and disappear without the subject noticing.) We discovered that change blindness is limited to inanimate objects. We found, as predicted, that animals are treated differently by visual attention.
In this paradigm, the only task subjects are given is to detect changes, so they are free to follow their own inclinations in attending to different entities in photos of complex natural scenes. Our experiments show that they are faster and more accurate at noticing changes in animals (and humans) relative to changes in plants, buildings, tools, vehicles and other artifacts. For example, changes to a small nonhuman animal at the periphery of a complex natural scene are detected faster and more accurately than changes to a large building at a scene’s center. It is a remarkable effect, once one has experienced it for oneself.
A series of control experiments show that the attentional advantage found for animals is not due to lower-level visual features, expectation of motion, task demands, or how interesting the targets are. Instead, the monitoring system responsible for this effect appears to be category-driven, that is, it is automatically activated by any target the visual recognition system has categorized as an animal (nonhuman or human). This effect is due to monitoring, not fast recognition: Research by the Thorpes shows that vehicles (for example) are recognized as such as quickly as animals are, but we find that only animals are monitored by attention in an ongoing fashion. Previously discovered principles guiding visual attention do not center around high-level category membership.
Phylogenetic shaping
These studies were designed to evaluate whether the effect we predicted and found originates in ontogeny or phylogeny. Humans might have acquired an animal monitoring system as an expertise derived from experience or training during ontogeny. Or, as we hypothesized, it might have been built into visual attention because of its benefits over evolutionary time, regardless of its current utility. We tested between these alternatives by experiments using targeted contrast classes. Vehicles were a particularly important contrast for two reasons: (i) Experiments by the Thorpes show that vehicles are recognized as quickly as animals are, but attentional monitoring has a different function and should be governed by a different process. (ii) Ontogenetically, monitoring sudden changes in the states and locations of vehicles is a highly trained skill of life-and-death importance to our car-driving, street-crossing subjects, but it was of no importance phylogenetically. In contrast, monitoring changes in animals was important phylogenetically, but monitoring pigeons and squirrels is merely a distraction in modern cities and suburbs. If the ontogenetic expertise hypothesis were true, one would expect people to develop a category-specialized system for monitoring vehicles, such that changes to vehicles are detected as well as—or, indeed, better than—changes to nonhuman animals. But we show that the reverse is true: Speed and accuracy at noticing changes was far greater for animals—even nonhuman ones—than for vehicles. In short, animal monitoring appears to result from an evolved program analogous to the appendix: there because it was adaptive in our evolutionary past, but relatively useless now.
Upper boundary on expertise
These studies also offered an opportunity to establish an upper bound on how sensitive visual attention is to training or expertise (at least for higher-level categories). For this purpose, it was important to include humans as a contrast class even though humans as a category confound both potential origins for attentional biases: Attending to humans would have been very important phylogenetically—but also, ontogenetically, humans are immersed from infancy in immense numbers of important transactions with other humans, which could have driven the acquisition of human-oriented attentional expertise without invoking any evolved bias towards humans. Indeed, our subjects have many orders of magnitude more experience with the human species than they do with taxa such as birds, turtles, insects, or African mammals. By comparing the performance of subjects on humans versus other species, we can evaluate the effect of all of this additional exposure on attention to humans in contrast to far less often experienced species. For the sake of argument, the assumption that all of the performance advantage accruing to humans is due to experience (and not some built-in bias) allows us to estimate the maximum possible upper bound of the effects of orders of magnitude more training with humans. Yet, the differences between attention to humans and to other species are marginal at best, suggesting that these systems are remarkably insensitive to training by exposure rates that differ by orders of magnitude.
Are the results novel and unexpected?
Yes: Indeed, looking for—and finding evidence of—category-specific attentional processes represents a significant departure from how the architecture of attention is usually conceptualized. Most research on visual attention has explored how attention is deployed in response either to lower-level features (color, intensity, orientation, contrast) or to information that is task-relevant or personally relevant given a volitionally chosen goal. Very few studies have considered the possibility that there are evolved systems designed to deploy attention in response to particular categories of information. The exceptions are a handful of studies of attention to human faces, human gestures, and stylized human outlines (silhouettes and stick figures) on a blank background. These studies have provided evidence of category-specific attentional processes for human stimuli. They cannot, however, address whether these processes arise from ontogenetic expertise or reflect the reliable development of a species-typical evolved mechanism because (1) subjects interact with other humans all the time, making human-derived stimuli a frequently encountered stimulus class, and (2) attention to other humans was important for accomplishing many goals and tasks now as well as ancestrally. In contrast, we were able to address this issue by examining attention to nonhuman animals, comparing it to attention to humans and to vehicles.
No other study has tested for category-specific attention to nonhuman animals—or indeed, to any category other than humans. Our experiments present evidence of category specific attention that extends to mammals, reptiles, birds, and insects, even when they are embedded in a complex natural scene. (Indeed, because our study uses natural scenes rather than isolated faces or gestures, our results also contribute to the study of category-specific attention to humans: we show that human figures command attention even when they are small, peripheral, minor elements of a photo with faces occluded).
Current approaches to attention often refer either to “salience” defined by integration of low level features or use intuitive notions of what is “informative”. This research helps cash out what the visual system finds “informative”—there are mid-level principles (the system finds animals salient or informative), not just high level task-relevant dimensions or low-level salience.
Relevance to different fields
The approach and results we present are a sharp departure from traditional approaches to visual attention, and of broad relevance to many different fields—behavioral ecologists, vision scientists, attention researchers, cognitive neuroscientists, cognitive developmentalists, neuroscientists, artificial intelligence researchers, and evolutionary biologists. More specifically:
- The discovery of category-specific attentional mechanisms may change thinking not only among vision and attention researchers, but also among the many artificial intelligence researchers who use research on attention to create machine vision—the idea of mid-level category-specific attention is not part of current AI approaches, but probably should be.
- Although attention researchers have rarely searched for category-specific mechanisms, this topic has been of interest in many other areas of the cognitive sciences and neuroscience. Cognitive neuroscientists have found category-specific semantic memory systems with dissociations for animate versus inanimate objects (these inspired our choice of categories to test), and cognitive developmentalists have found inference systems in infants and children that make sharp distinctions between animate versus inanimate objects (and even between humans and other animals).
- Behavioral ecologists and evolutionary biologists search for adaptive neurocomputational systems in many species. By showing evidence of an attentional system in humans specialized for attending to nonhuman animals, these studies should spawn the search for similar category-specialized attentional monitoring systems in other species where predator-prey interactions are an important selection pressure.
Intelligent Design?
This research also represents another demonstration of a contested point: That consideration of how humans evolved can inform various subfields of neuroscience and psychology. The very idea that humans evolved has come under legal siege in the U.S. during the last several years. It is important to continue to demonstrate that humans no less than other species show significant evidence of being the organized product of natural selection—and in subtle, unexpected ways not easily explained by blank-slate learning or “i